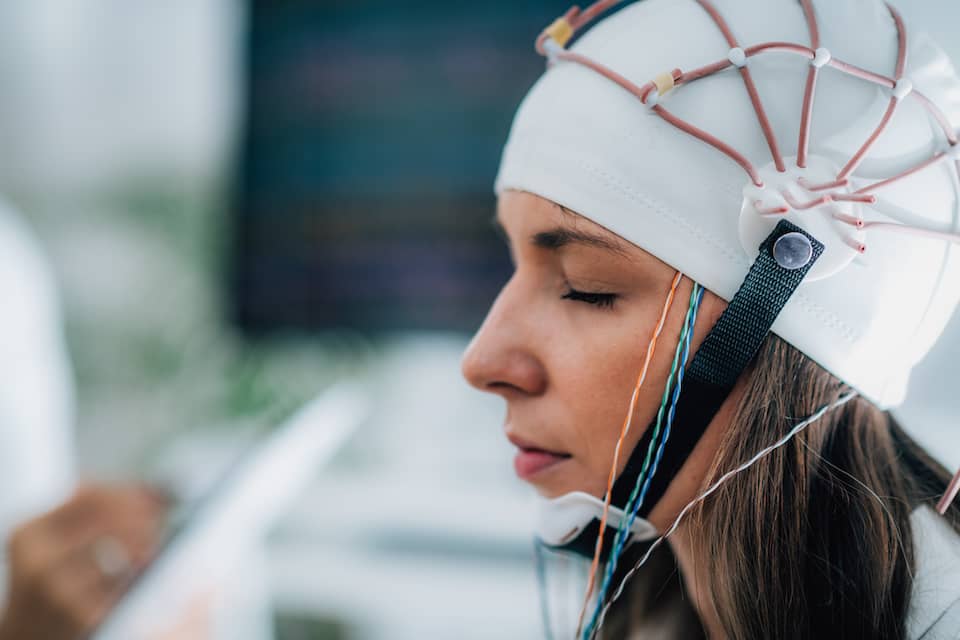
Jian Hu, France W Fung, Marin Jacobwitz, Darshana S Parikh, Lisa Vala, Maureen Donnelly, Alexis A Topjian, Nicholas S Abend, Rui Xiao
Published in Seizure
Electroencephalographic seizures (ES) occur in 10-40% of children with acute encephalopathy who undergo continuous EEG monitoring (CEEG), increasing evidence indicates that high ES exposure is associated with unfavorable neurobehavioral outcomes, and ES are often treatable with anti-seizure medications. However, given CEEG is resource-intense, a clinical prediction tool that enabled evidence-based targeting of CEEG resources to patients most likely to experience ES would be of great clinical value. In this study, we investigated on whether machine learning techniques would enhance our ability to incorporate key variables into a parsimonious model with optimized prediction performance for ES prediction in critically ill children. We analyzed data from a prospective observational cohort study of 719 consecutive critically ill children with encephalopathy who underwent clinically-indicated continuous EEG monitoring (CEEG). We implemented and compared three state-of-the-art machine learning methods for ES prediction: (1) random forest; (2) Least Absolute Shrinkage and Selection Operator (LASSO); and (3) Deep Learning Important FeaTures (Deep- LIFT). We developed a ranking algorithm based on the relative importance of each variable derived from the machine learning methods.