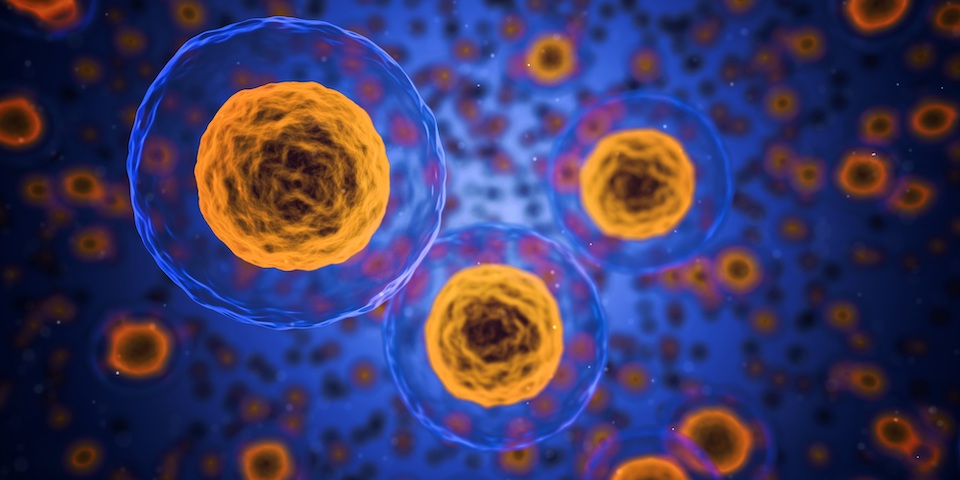
The advent and rapid development of single-cell technologies have made it possible to study cellular heterogeneity at an unprecedented resolution and scale. Cellular heterogeneity underlies phenotypic differences among individuals, and studying cellular heterogeneity is an important step toward our understanding of the disease molecular mechanism. Single-cell technologies offer opportunities to characterize cellular heterogeneity from different angles, but how to link cellular heterogeneity with disease phenotypes requires careful computational analysis. This project proposes to develop computational strategies for the analysis of single-cell data for various purposes, including batch effect removal, missing gene imputation, cell type identification and cell differentiation trajectory. We also describe how single-cell data can be integrated with bulk tissue data and data generated from genome-wide association studies.
In collaboration with: Mingyao Li, Rui Xiao